
The smart camera trap, FASTCAT-Edge, developed by DynAikon in the Cos4Cloud framework, improves animal detection compared to commercial camera traps. It does so by avoiding Passive Infra-Red (PIR) motion sensors that require body heat to trigger and usually record empty images and using artificial intelligence instead. This has been the main conclusion of a recent scientific article published by Ecological Informatics (ScienceDirect) and authored by the DynAikon members and FASTCAT-Edge developers, who are also part of the Cos4Cloud team: Miklas Riechmann, Ross Gardiner, Kai Waddington, Ryan Rueger, Frederic Fol Leymarie and Stefan Rueger.
In particular, FASTCAT-Edge integrates a type of artificial intelligence known as deep neural networks into its system. This technology significantly reduces the delay from animal motion detection to the image being taken. It can also differentiate between animal motion and other movements, such as leaves falling from a tree.
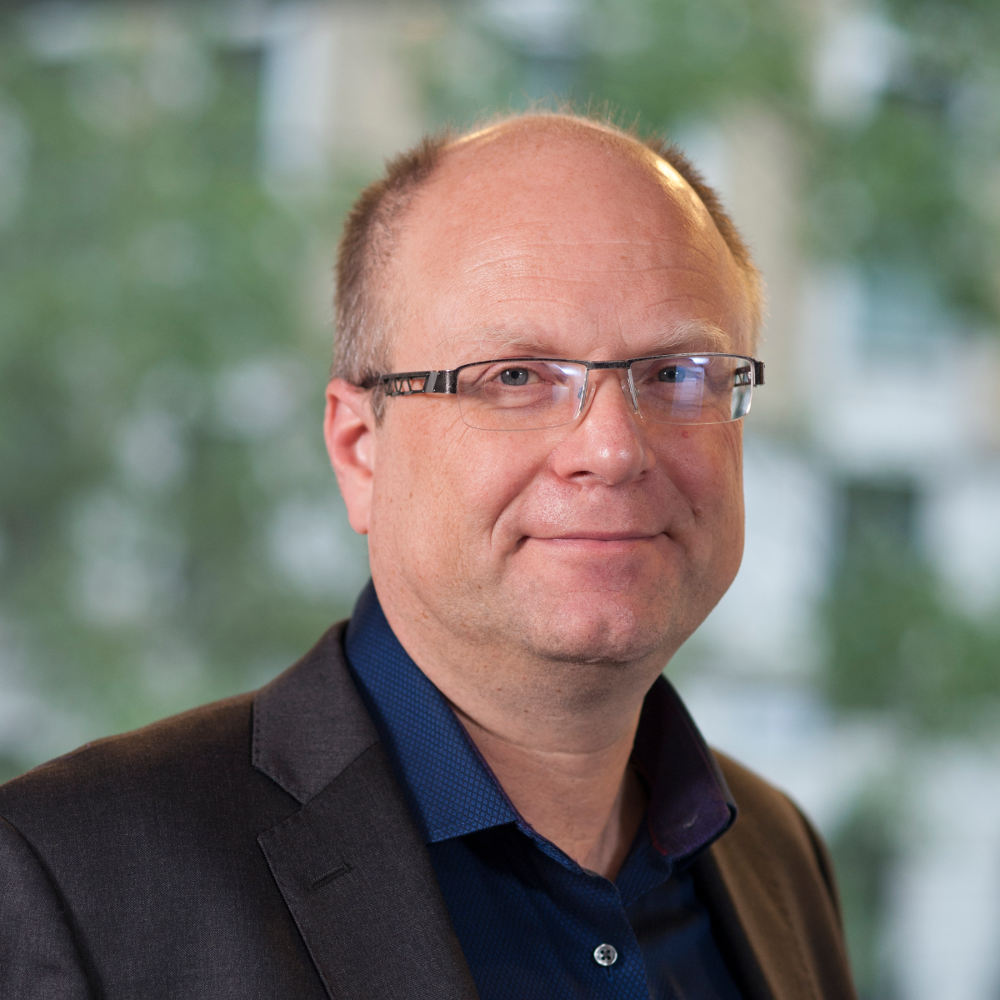
“Some commercial camera traps have a long shutter delay (0.5 s to 1 s), so an animal might have left the camera’s field of view when it takes the picture. FASTCAT-Edge solves this problem and significantly reduces the capture of “empty” images”.
Stefan Rueger, co-director of DynAikon, professor at The Open University’s Knowledge Media Institute and Cos4Cloud member.
FASTCAT-Edge can also detect small animals, sometimes missed with standard commercial camera traps because they need a minimum heat threshold to be triggered. It also improves fast animal detection because there is almost no delay from motion being detected to the image being taken.
Another advantage of this camera trap system is that it can capture animals at the same temperature as the background—for example, wet animal skin, animals in a hot environment or fish in water. Therefore, users can use this camera trap for maritime environments. “Moreover, it is possible to create a long-range camera trap, e.g., using a wide lens to monitor birds or detecting insects using macro lenses”, adds Stefan Rueger. In addition, FASTCAT-Edge’s cutting-edge technology considers animals to select the “best” frame as the key image of the observation; this is based on the use of series of frames having triggered a movement detection event.
FASTCAT-Edge, a do-it-yourself camera trap connected to the citizen science community
FASTCAT-Edge consists of an affordable, compact, low-power Raspberry Pi computer (RPi) that anyone can build following the open guideline. Moreover, the software that runs FASTCAT-Edge integrates easily with the sibling service FASTCAT-Cloud, which allows you to identify the species in your observations through AI and upload your observations to citizen observatories such as iSpot and through interfaces such as the SensorThingsAPI plus.
“This article also lays the foundation for automated camera trapping being capable of generating trustworthy datasets, and this could contribute to improving data quality in citizen science”, concludes Stefan.
If you want to know more about FASTCAT-Edge visit:
Reference article
Riechmann, M., Gardiner, R., Waddington, K., Rueger, R., Leymarie, F. F., & Rueger, S. (2022). Motion vectors and deep neural networks for video camera traps. Ecological Informatics, 69. https://doi.org/10.1016/j.ecoinf.2022.101657