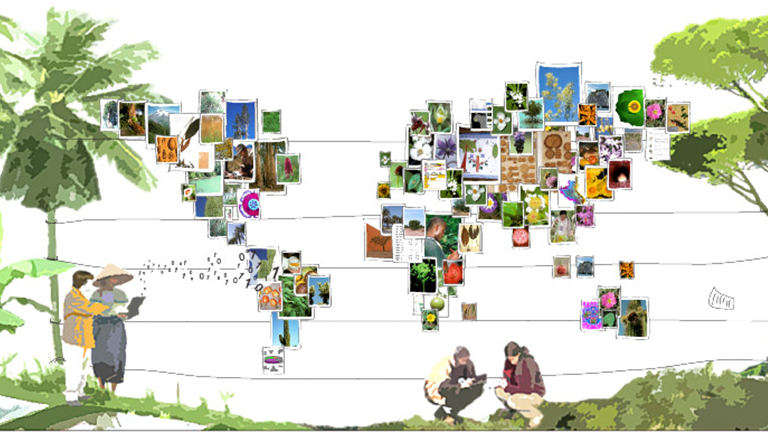
A major obstacle to better management of biodiversity is what is sometimes called the taxonomic gap: the fact that very few people are able to identify plants and animals with precision. Indeed, the scientific name of a species is the entry point for accessing information about this species. It is also an essential element for many processes related to its preservation, such as the modeling of its distribution, the determination of its conservation status, or its integration in implementing new agroecological methods that are more environmentally friendly.
In less than ten years, Artificial Intelligence (AI) has enabled lightning progress in this field. Until the 2010s, only specialists were able to identify plants and animals with precision by relying on determination keys whose language is very complex and almost incomprehensible for a neophyte. Currently, an AI system like the one of Pl@ntNet, developed in the European project Cos4Cloud framework, can recognize more than 35K species with an accuracy close to that of the best experts for most of them.
However, this breakthrough was not achieved in two days. Before the AI boom, state of the art in automated identification consisted mainly of developing descriptor extraction algorithms. These descriptors, such as the color of the petals or the leaf texture, were defined upstream by specialists. The recognition process then consisted mainly of comparing the descriptors of a query image with the descriptors extracted from the images in the database.
With the rise of machine learning, the big difference is that the algorithm learns which visual patterns are the most discriminating without prior knowledge. And the great strength of an AI is that it can do this learning in a few days on tens of thousands of species without memory problems!
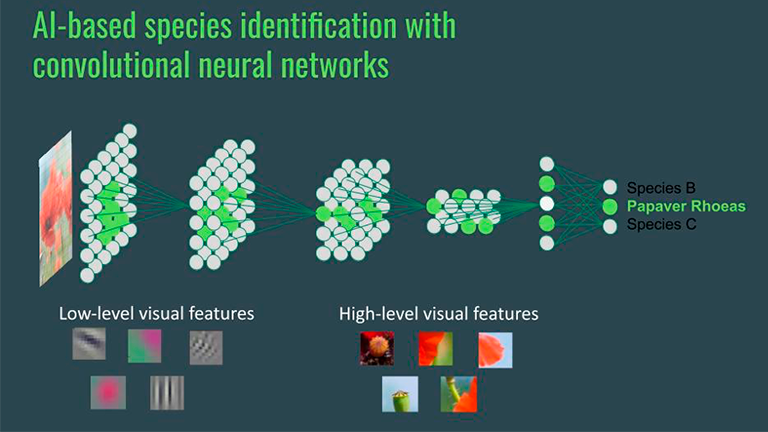
This high learning capacity, however, does not mean that an AI model does not need teachers. An AI like the one integrated into Pl@ntNet has tens of thousands of teachers who provide it with training images of the species it recognizes. These images must be numerous and varied enough to contain the most relevant information. They must also include as few errors as possible so that the AI does not make mistakes and does not lead other users into the same mistakes. To achieve this quality requirement, it is essential to develop efficient tools to collect and process species name proposals from users.
In the Cos4Cloud project, we are developing a web application called Cos4Bio that allows experts and informed amateurs to review data from several European citizen observatories. Most of these observatories: Artportalen, iSpot, Natusfera and Pl@ntNet, incorporate strategies for weighting their contributors according to their expertise.
AI-GeoSpecies: the next step to predict species distribution
In addition to recognizing plants and animals from photos, AI systems will also soon be able to tell you which species you can observe in a given place before you even go there! To do this, they will rely on species occurrence data collected by citizen observatories during the last decade. But this is not enough. Despite their large numbers, they are far from covering the whole territory, especially if we want to reach a high geographical precision. Moreover, we are in a very changing world and the species present ten years ago may be different from those present today.
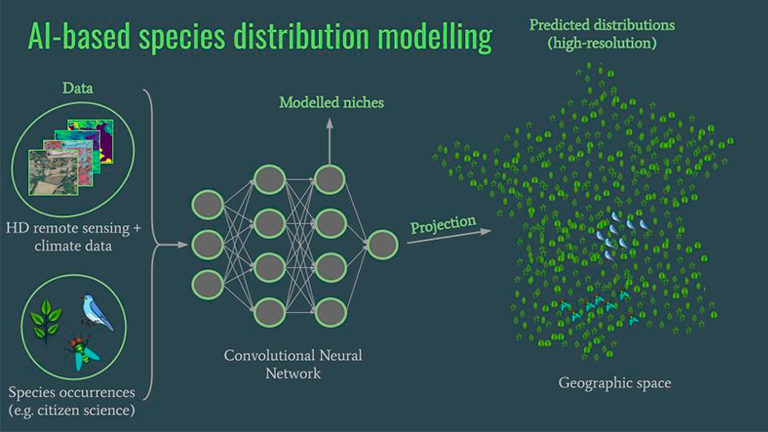
The AI system we are working on in Cos4Cloud, AI-GeoSpecies, uses complementary data such as satellite images or aerial views at a very accurate spatial resolution to predict the species present better. By combining these data with the data coming from citizen observatories, the AI can learn to recognize the habitats in which species live and thus predict their presence, in places where they have not been observed in the database. Eventually, this type of AI will allow us to build maps of species distribution at very accurate scales of about ten meters or less! They will thus enable everyone to act locally with a much better knowledge of local biodiversity, whether he is a mayor of a town, a land-use planning professional, or a simple citizen.